Sync4D: Video Guided Controllable Dynamics for Physics-Based 4D Generation
- Zhoujie Fu *,1
- Jiacheng Wei *,1
- Wenhao Shen 1
- Chaoyue Song 1
- Xiaofeng Yang 1
- Fayao Liu 2
- Xulei Yang 2
- Guosheng Lin 1
4D generation on various types of objects
Sync4D enables high-quality 4D generation while maintaining shape consistency and motion correctness, guided by casually captured videos.
Abstract
In this work, we introduce a novel approach for creating controllable dynamics in 3D-generated Gaussians using casually captured reference videos. Our method transfers the motion of objects from reference videos to a variety of generated 3D Gaussians across different categories, ensuring precise and customizable motion transfer. We achieve this by employing blend skinning-based non-parametric shape reconstruction to extract the shape and motion of reference objects. This process involves segmenting the reference objects into motion-related parts based on skinning weights and establishing shape correspondences with generated target shapes. To address shape and temporal inconsistencies prevalent in existing methods, we integrate physical simulation, driving the target shapes with matched motion. This integration is optimized through a displacement loss to ensure reliable and genuine dynamics. Our approach supports diverse reference inputs, including humans, quadrupeds, and articulated objects, and can generate dynamics of arbitrary length, providing enhanced fidelity and applicability. Unlike methods heavily reliant on diffusion video generation models, our technique offers specific and high-quality motion transfer, maintaining both shape integrity and temporal consistency.
Sync4D = Reference Shape and Motion + Object Parts Matching + Physics-Based Motion Driven
Sync4D processes a reference video to derive a canonical shape and a bone-based motion sequence through reconstruction techniques. Meanwhile, given a text prompt or image prompt, we generate a 3D Gaussian object through diffusion models. The framework matches motion-related parts from the reconstructed shape to the generated shape and transfers the motion. This motion information is then initialized into the velocity physical signals. We employ a triplane representation to produce a delta velocity field to adjust physical signals. The velocity field for each part of the target is optimized using the differentiable Material Point Method (MPM) simulation. To ensure fidelity to the original, a displacement loss is designed to reduce cumulative errors and ensure plausible motions.
Results gallery
We present a gallery showcasing our results in transferring motions to general 3D static object from human, quadruped, and articulated object.
Ref Video: Human
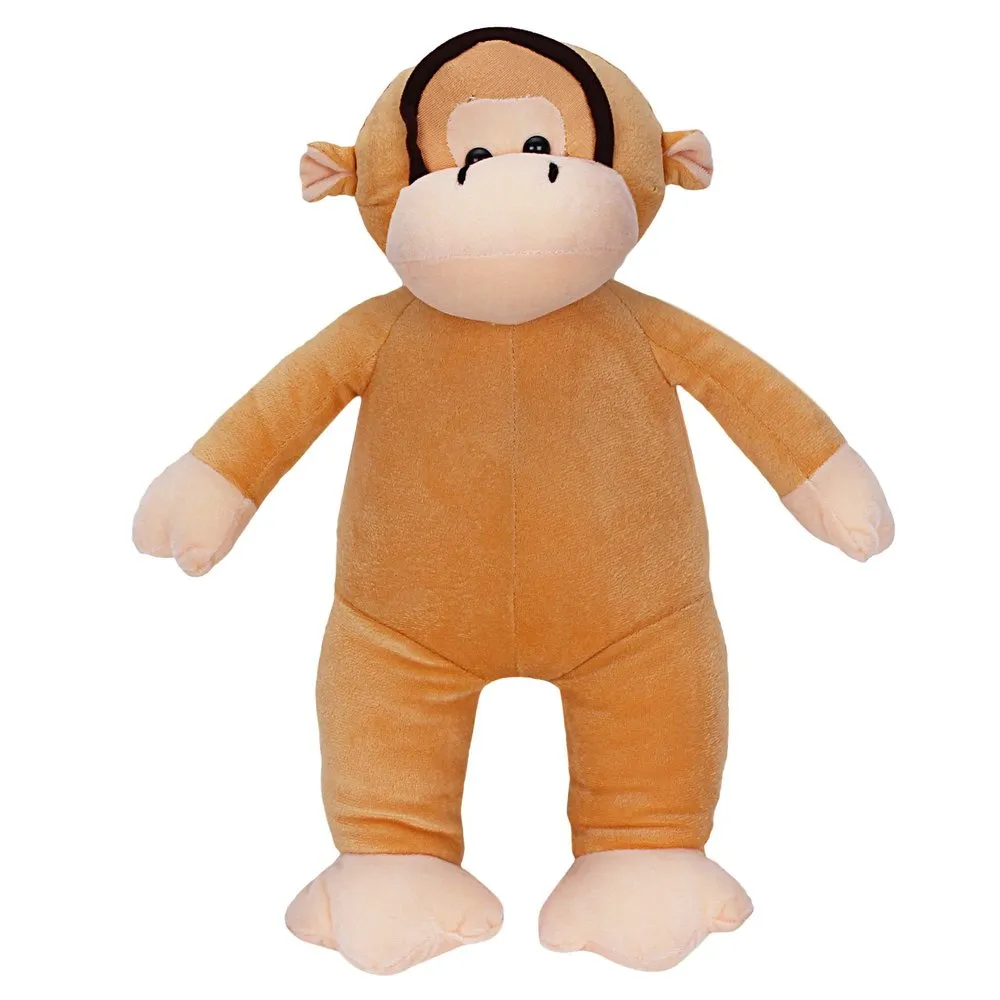
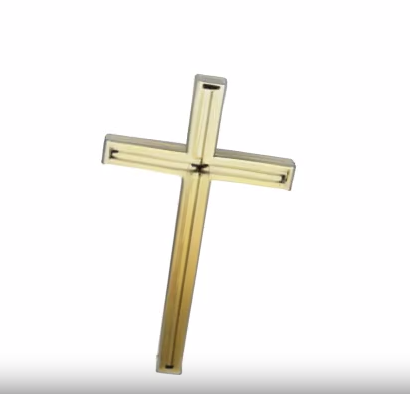
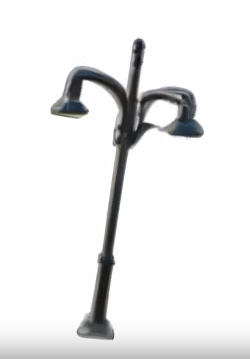
Ref Video: Cat
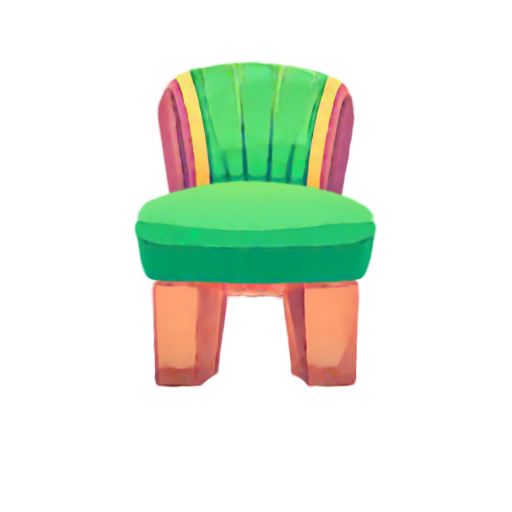
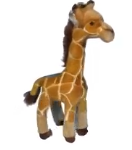
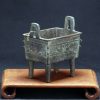
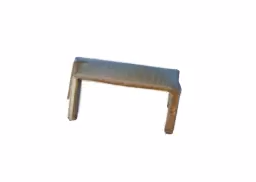
Ref Video: Laptop
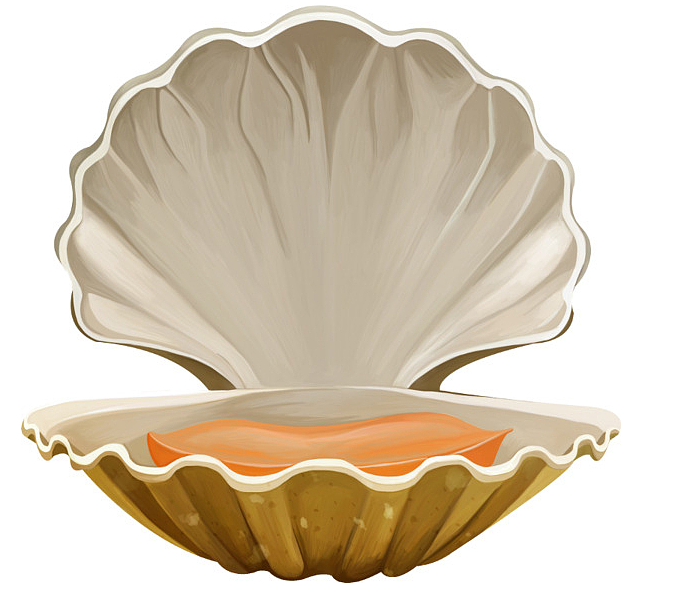
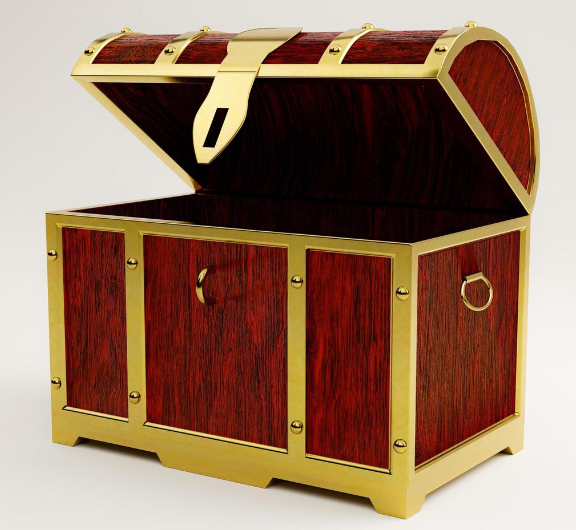
Citation
Acknowledgements
The website template was borrowed from PhysDreamer.